Kirchdoerfer T, Ortiz M (2016) Data-driven computational mechanics. Computer Methods in Applied Mechanics and Engineering 304:81-101, doi:10.1016/j.cma.2016.02.001.
Kirchdoerfer T, Ortiz M (2017) Data-driven computing with noisy material data sets. Computer Methods in Applied Mechanics and Engineering 326:622-641, doi:10.1016/j.cma.2017.07.039.
Ayensa-Jiménez J, Doweidar MH, Sanz-Herrera JA, Doblaré M (2018) A new reliability-based data-driven approach for noisy experimental data with physical constraints. Computer Methods in Applied Mechanics and Engineering 328:752-774, doi:10.1016/j.cma.2017.08.027.
Conti S, Müller S, Ortiz M (2018) Data-driven problems in elasticity. Archives in Rational Mechanics and Analysis 229:79-123, doi:10.1007/s00205-017-1214-0.
Nguyen LTK, Keip MA (2018) A data-driven approach to nonlinear elasticity. Computers & Structures 194:97-115, doi:10.1016/j.compstruc.2017.07.031.
Ayensa-Jiménez J, Doweidar MH, Sanz-Herrera JA, Doblaré M (2019) An unsupervised data completion method for physically-based data-driven models. Computer Methods in Applied Mechanics and Engineering 344:120-143, doi:10.1016/j.cma.2018.09.035.
Arridge S, Maass P, Öktem, O, Schönlieb CB (2019) Solving inverse problems using data-driven models. Acta Numerica 28:1-174, doi:10.1017/S0962492919000059.
Eggersmann R, Kirchdoerfer T, Reese S, Stainier L, Ortiz M (2019) Model-free data-driven inelasticity. Computer Methods in Applied Mechanics and Engineering 350:81-99, doi:10.1016/j.cma.2019.02.016.
Leygue A, Seghir R, Réthoré J, Coret M, Verron E, Stainier L (2019) Non-parametric material state field extraction from full field measurements. Computational Mechanics, doi:10.1007/s00466-019-01725-z.
Stainier L, Leygue A, Ortiz M (2019) Model-free data-driven methods in mechanics: material data identification and solvers. Computational Mechanics, doi:10.1007/s00466-019-01731-1.
5 lectures on: handling data with random properties in data-driven applications; using physical knowledge to improve data handling with example to data filling in missing data problems; introducing the concept of physically-informed machine learning with examples in the prediction of input-output relations in problems defined by PDE systems and in extracting internal state knowledge.
5 lectures on: variational approaches to distance-minimizing data-driven computational mechanics with applications in elasticity; Artificial-Neural-Network (ANN) assisted computational homogenization of experimental microstructures based on image recognition; hands-on training session on implementing ANN algorithms in an open-source machine-learning code.
5 lectures on: handling data with random properties in data-driven applications; using physical knowledge to improve data handling with example to data filling in missing data problems; introducing the concept of physically-informed machine learning with examples in the prediction of input-output relations in problems defined by PDE systems and in extracting internal state knowledge.
6 lectures on: basics of digital image correlation (DIC) and its resolution; numerical algorithms for the optical flow problem; the uncertainty / resolution compromise and regularization techniques; Finite Element Model Updating (FEMU) methods; data-driven identification (DDI) approach, formulation and implementation; hands-on session in DIC and DDI with applications to linear and non-linear elasticity.
6 lectures on: inverse imaging problems; variational regularisation models and partial differential equations; sparsity-promoting regularisation and total variation; applications to tomography, image segmentation, motion estimation and image fusion; mathematical analysis and numerical algorithms; machine learning approaches such as learned iterative reconstruction and learned regularisers with deep neural networks.
6 lectures on: distance minimizing data-driven algorithms; integrated mechanical design approach combining DDI+DDCM; importance sampling issues; hands-on session (DDI based DDCM for nonlinear elasticity); algorithmic strategies and data representation for history-dependent Data-Driven simulations; examples and illustration of the methodology in elasto-plasticity.
The registration fee is 600.00 Euro + VAT*, where applicable (bank charges are not included). The registration fee includes a complimentary bag, four fixed menu buffet lunches (on Friday upon request), hot beverages, downloadable lecture notes and wi-fi internet access.
Applicants must apply at least one month before the beginning of the course. Application forms should be sent on-line through the following web site: http://www.cism.it. A message of confirmation will be sent to accepted participants. Applicants requiring assistance with the registration should contact the secretariat at the following email address cism@cism.it.
Applicants may cancel their course registration and receive a full refund by notifying CISM Secretariat in writing (by email to cism@cism.it) no later than two weeks prior to the start of the course.
Cancellation requests received during the two weeks prior to the start of the course will be charged a 50.00 Euro handling fee. Incorrect payments are also subject to a 50.00 Euro handling fee.
A limited number of participants from universities and research centres who are not supported by their own institutions can be offered lodging and/or board, if available, in a reasonably priced hotel or student guest house.
Requests should be sent to CISM Secretariat by February 20, 2020 along with the applicant's curriculum and a letter of recommendation by the head of the department or a supervisor confirming that the institute cannot provide funding. Preference will be given to applicants from countries that sponsor CISM.
Information about travel and accommodation is available on the web site www.cism.it, or can be mailed upon request.
* Italian VAT is 22%.
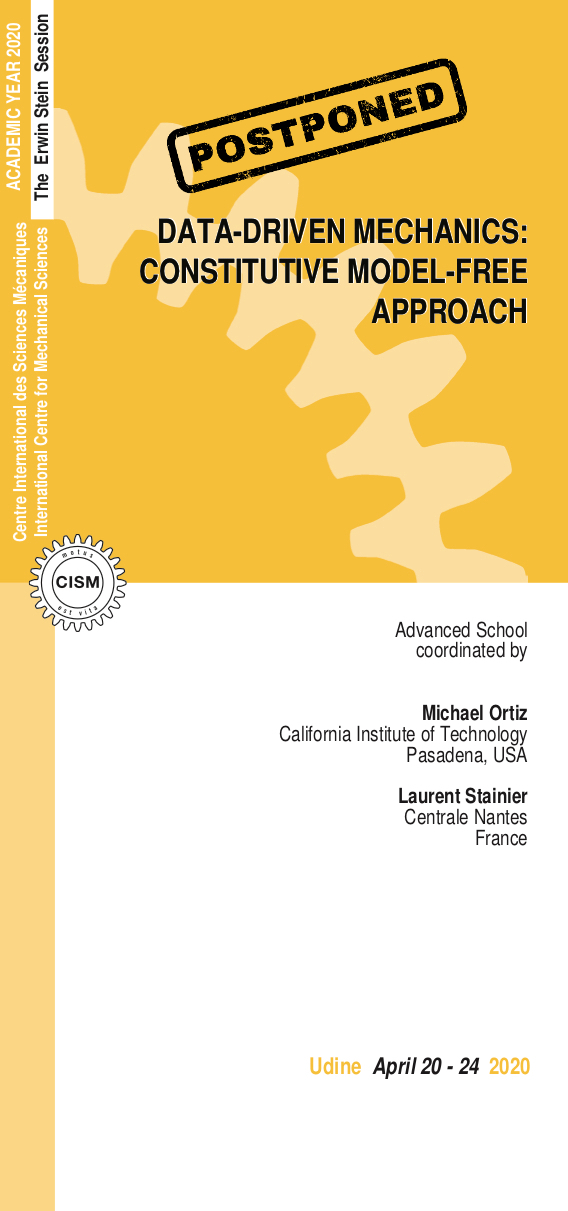